How Poor Spelling Causes Duplicate Data - a Case Study
AICA has long understood that spelling accuracy is crucial for maintaining high-quality, reliable data. Poor spelling can cause significant issues in product data, particularly in creating duplicate entries.
This case study highlights the impact of misspellings on data integrity and operational efficiency, focusing on how they lead to duplicate data entries.
The Issue with Misspellings
When product data is misspelt, it can prevent the correct entry from being found during searches or data analysis. This oversight often leads to the same product being re-entered into the dataset under different, incorrect spellings. Consequently, this results in duplicate entries that clutter the dataset and complicate data management.
Example From Our Recent Project
In our cleansing exercise, we encountered a common issue in the MRO product data environment. A critical component, such as a Hydraulic Valve, was misspelt multiple times in the database:
– Hydraulic Valv
– Hydraulic Valvue
– Hidraulic Valve
Each misspelling was treated as a separate item in the MRO dataset, leading to inconsistencies and inefficiencies.
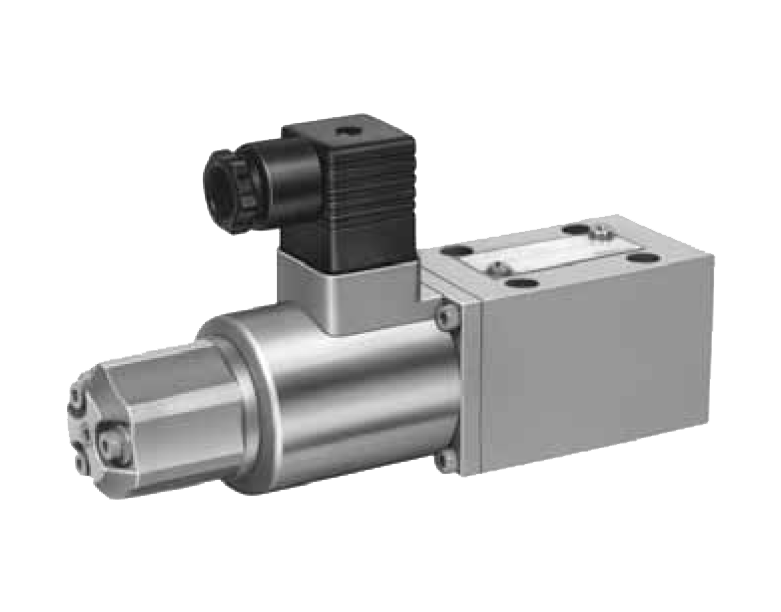
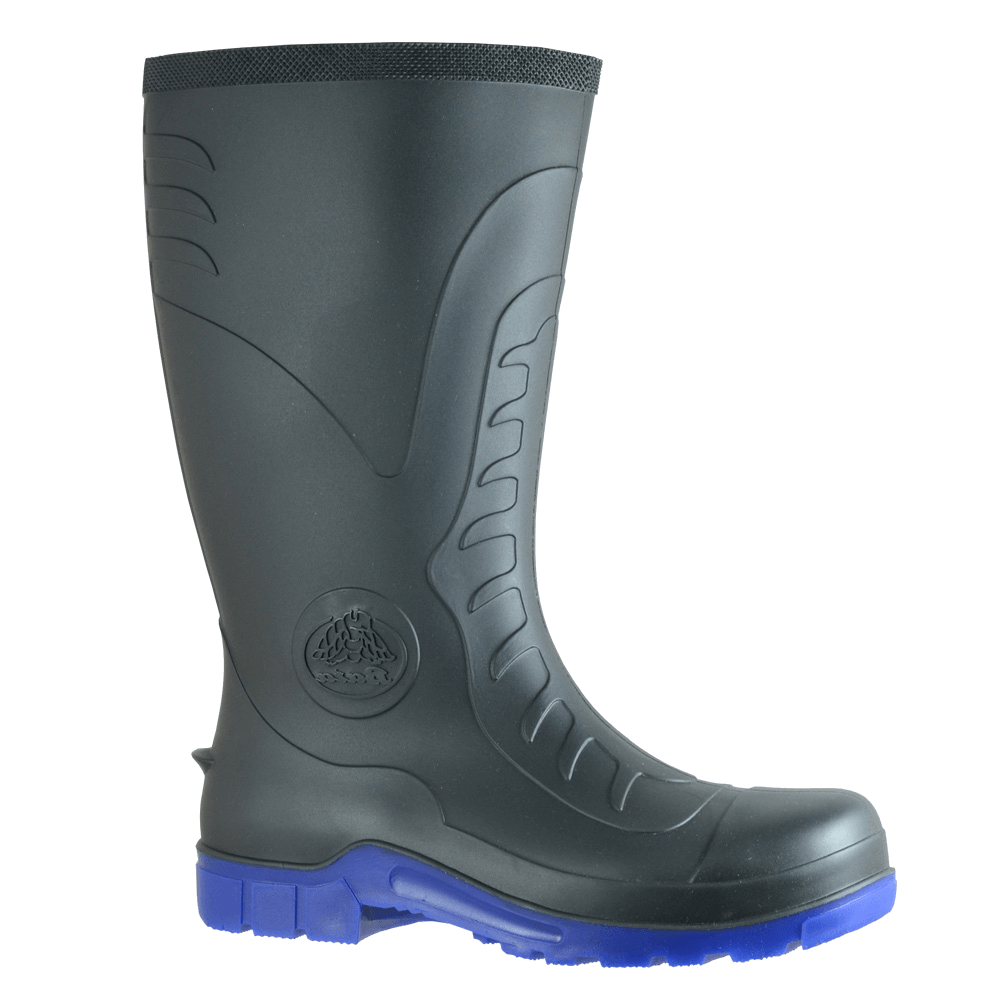
During the standardisation and normalisation procedures, we encountered multiple entries of the same items entered slightly differently. “Gumboots” appeared multiple times in different formats throughout the dataset.
– GUMBOOT
– GUMBOOTS
– GUM BOOT
– GUM BOOTS
Each item was treated as a seperate item in the MRO dataset, leading to a vast amount of duplicates going unnoticed.
When the organisation’s technicians searched for “Hydraulic Valve” and “Gumboots” to check inventory or place an order, the misspelt and non standardised entries did not appear in the search results. This led technicians to believe that the items were not already in the system, prompting them to re-enter the component’s information. Consequently, this resulted in multiple duplicate entries for the same hydraulic valve and the same pair of gumboots, each with a slightly different spelling.
Data Cleansing Summary
Initial Dataset Size
100%Spelling Errors Corrected
12.75%Duplicates Identified and Removed
27.84%Remaining Items after Deduplication
72.16%Process
Language Correction
Corrected spelling errors equal to 12.75% of the initial dataset
Standardisation & Normalisation
Standardised and normalised text data to a uniform format
De-duplication
Identified and removed duplicates equal to 27.84% of the initial dataset
Data Quality
Achieved a more consistent and reliable dataset with remaining items equal to 72.16% of the initial dataset
The Impact of Duplicates
Duplicates in MRO data creates several issues:
- rInaccurate Inventory Counts: Multiple entries for the same part can lead to overstocking or stockouts, as the inventory system might count them as separate items.
- Complicated Data Analysis: Duplicate entries skew maintenance schedules and spend analysis, making it difficult to derive accurate insights.
- Inefficient Operations: Managing and reconciling duplicate entries consumes time and resources, reducing overall operational efficiency.
- Increased Error Rates: Automated systems relying on accurate data struggle with inconsistencies caused by duplicates, leading to higher error rates.
Steps to Prevent Duplicate Entries
Implement Data Governance Policies
Define data standards, ownership, and processes for data management across the organisation to ensure consistency and accuracy.
Conduct Regular Data Audits
Regularly audit data to identify and rectify errors, maintaining ongoing data integrity.
Utilise Data Cleansing Tools
Use tools like AICA to automate error correction, saving time and reducing manual effort.
Standardise Data Entry
Use predefined formats for data input to minimise the risk of errors and ensure uniformity.
Conclusion
Poor spelling can lead to significant issues in product data, primarily through the creation of duplicate entries. By prioritising spelling corrections and data normalisation, we achieved a more streamlined, reliable dataset, enhancing overall data integrity and operational efficiency.
This project highlights the importance of meticulous attention to detail in data management, setting a strong foundation for future success.
Improve your product data quality by addressing spelling errors and duplicates. Get your free product data quality report today!